In a rapidly evolving world, technology stands at the forefront, dictating the pace of innovation and redefining what’s possible. Among the many revolutionary advances shaping our future, artificial intelligence (AI) and machine learning (ML) hold a special place, promising to transform industries, simplify tasks, and enrich the human experience.
For tech enthusiasts and students eager to keep their finger on the pulse of progress, it’s crucial to stay abreast of the most recent AI and ML developments. This in-depth exploration uncovers five of the most exciting advancements that not only shed light on where AI and ML are headed but also provide a roadmap for potential applications and emerging trends.
1. Federated Learning- AI’s Leap Towards Data Privacy and Security
Federated Learning has emerged as an ingenious solution to the perennial challenge of data privacy and security. With privacy concerns at an all-time high, this approach allows AI to be trained across multiple decentralized edge devices (such as smartphones) without the need for data to be stored or shared in a central repository.
Imagine that instead of sending your personal data to a central server for processing, your device learns locally from this data and only shares minimal insights with the system that aggregates knowledge across devices. The implications are remarkable; not only does Federated Learning protect sensitive information, but it also results in more personalized and effective AI models.
This paradigm shift in model training is already finding applications in healthcare, finance, and beyond. Health monitoring apps, for instance, can adapt to individual needs without compromising patient privacy, while financial institutions can improve fraud detection algorithms without exposing transaction details.

2. Transformer Architectures- Revolutionizing Natural Language Processing
Natural Language Processing (NLP) is taking a quantum leap forward with the rapid adoption of Transformer architectures. Originally designed for machine translation tasks, Transformers—an innovation popularized by Google’s ‘BERT’ and OpenAI’s ‘GPT’ models—have showcased their remarkable versatility and applicability to a wide array of NLP problems.
These architectures have revolutionized NLP by allowing machines to understand and generate human language with previously unseen accuracy. By leveraging self-attention mechanisms, Transformers can process entire sentences in context, leading to significantly improved language understanding and generation capabilities.
In practical terms, this means chatbots that can hold more natural conversations, search engines that can better understand user queries, and a new breed of content generation tools capable of crafting human-sounding prose. The impact of Transformer architectures extends well beyond enhancing customer interactions; they’re also at the heart of important research in fields like journalism, where they are used to supplement news writing.
3. Continuous Learning Frameworks- AI That Never Stops Improving
One of the defining characteristics of traditional AI systems is their static nature; they are trained on fixed datasets and don’t learn from new information or experiences after deployment. Continuous Learning Frameworks seek to change this by enabling AI models to evolve over time, leveraging new data to improve their performance continually.
This approach is particularly advantageous in dynamic environments where data distributions can change rapidly, requiring real-time adaptations. Autonomous vehicles, for instance, rely on continuous learning to adapt to new traffic patterns and conditions. In the retail sector, AI that learns continuously can respond to shifting consumer preferences with better product recommendations.
Continuous learning systems also address the challenge of ‘catastrophic forgetting,’ where AI systems that learn sequentially forget previously learned information. By building on existing knowledge, these frameworks ensure that new learning complements prior experiences, resulting in more stable and adaptable AI.
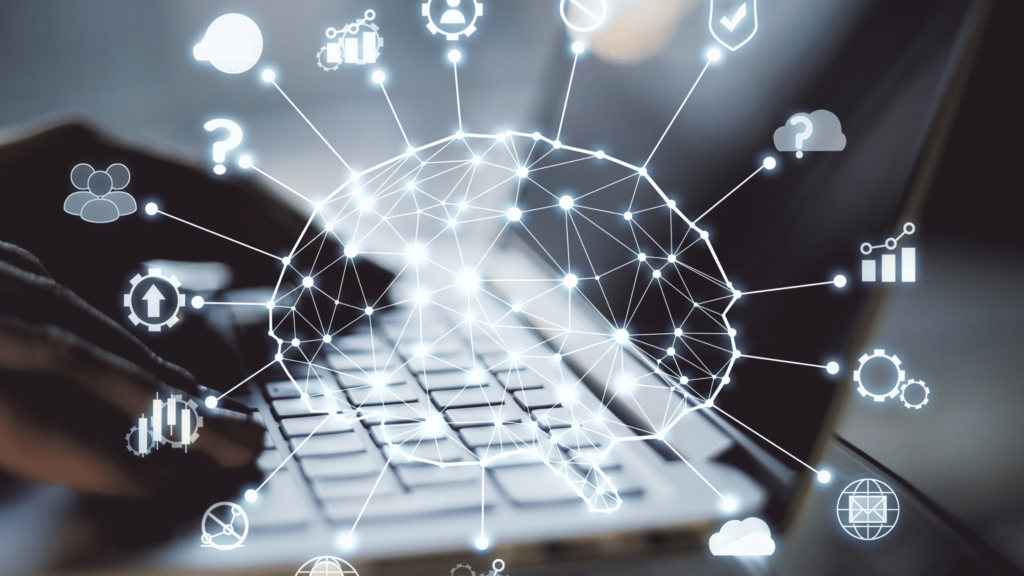
4. AI-Driven Personalization at Scale- The Next Frontier for Consumer Engagement
In the digital age, personalized experiences are becoming the norm rather than the exception. Yet, achieving personalization at scale poses a significant challenge, one that AI is uniquely positioned to tackle. With advancements in AI-driven personalization, companies can create individualized customer journeys at a level of detail and complexity that would be unmanageable without automation.
This next frontier in consumer engagement harnesses ML to analyze vast amounts of data, including user behavior, historical interactions, and real-time feedback, to deliver tailor-made content, product recommendations, and marketing messages. The sophistication of these models allows for a level of precision that was once the realm of science fiction—predicting customer needs and desires before they’re even articulated.
The applications of AI-driven personalization are ubiquitous. E-commerce platforms can enhance the customer shopping experience with recommendations that reflect the latest trends and personal preferences. Streaming services can suggest content based not only on viewing history but also on current mood and context. This form of AI not only drives engagement but also fosters brand loyalty through thoughtful personalization.
5. Reinforcement Learning for Real-World Optimization
Reinforcement Learning (RL) is poised to enter new realms of applicability, particularly in the realm of real-world optimization. Unlike supervised learning, where the AI model learns from labeled input-output pairs, and unsupervised learning, which derives structure from data, RL is about learning to make sequences of decisions.
RL’s foundation in behavioral psychology and its proven ability to solve complex, uncertain problems make it ideal for domains where trial and error are intrinsic to finding optimal solutions. This includes robotics, industrial control systems, and autonomous decision-making systems.
In the context of robotics, RL enables robots to learn how to perform intricate tasks by themselves, such as assembling different parts in a factory setting. Industrial control systems can optimize energy usage or manage complex supply chains more effectively, adapting to the dynamic nature of these environments. Autonomous decision-making systems driven by RL can be found in autonomous drones, which learn to operate safely and efficiently in changing airspace conditions.
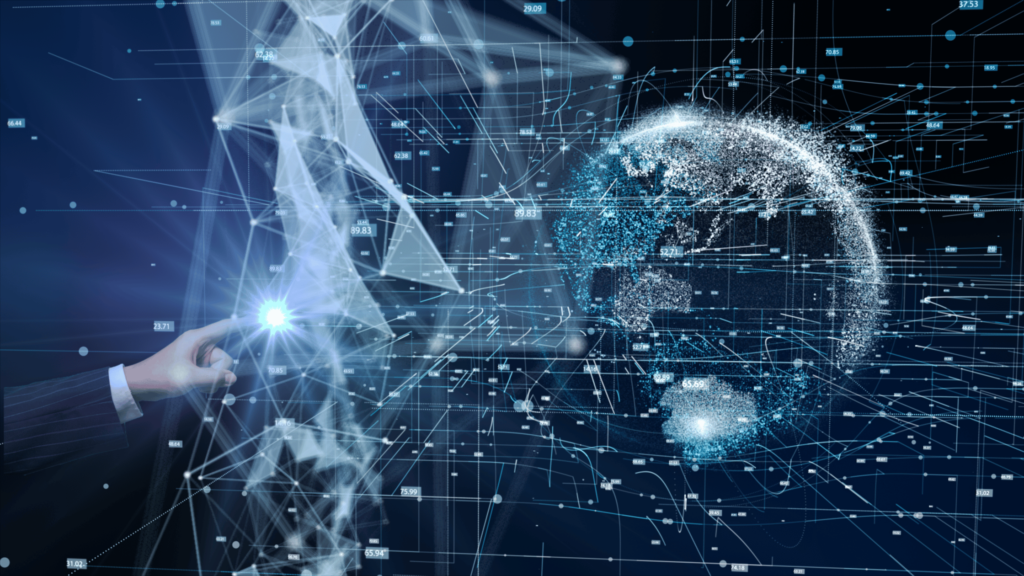
In Conclusion
The rapid progression of AI and ML technologies is reshaping the landscape of what is possible, not just in the tech industry, but across all sectors of the global economy. From revolutionizing data privacy to enabling highly-personalized consumer experiences, these technologies are not just advancing—they’re evolving to meet the most pressing needs of our time.
For students and professionals alike, understanding and keeping pace with AI and ML developments is not just an academic exercise—it’s a crucial step toward shaping a future where intelligent systems work alongside humans to tackle pressing challenges and unlock new opportunities. As these five cutting-edge developments demonstrate, the possibilities are endless, and the potential for innovation is boundless.